[Keywords] Genomic medicine, Precision medicine, Tumor microenvironment, Deep learning, Mathematical simulation, Quantum computing
Understand Life Phenomena and Diseases with Advanced Mathematical Science and Molecular Observation Technology
In our laboratory, we aim to understand the dynamics of diseases and immunity at the molecular level to overcome diseases such as cancer through the analysis of vast amounts of biomolecular data from many clinical specimens using mathematical/computational techniques like deep learning. It is expected that medical care will shift towards patient specific optimal therapies and drug dosages to treat and prevent disease. To do so, we need to develop new mathematical scientific methodologies based and analyze big data in human biomedical sciences, such as spatio-temporal omics molecular data, imaging data, and clinical information. In this way, we will discover new causes of disease and reconstruct quantitative networks of the relationships among these contributing factors to understand disease mechanisms as a whole system. As an example, by unraveling and quantitatively reconstructing the relationships of cells in the tumor microenvironment, including the immune system, we can predict the dynamics of response to treatment, side effects, and acquisition of resistance for each patient.
Understand Tumor-microenvironments
By investigating the relationship between cancer cell populations and their microenvironment, such as the immune system, we can model and predict response to treatment, side effects, and the acquisition of resistance on an individual basis (Figure 1-1). Recently, through the analysis of the whole genome sequence of liver cancer cells from 300 patients, we found a cluster with mutations within a novel cancer-related gene [1]. Patients in this cluster have a good prognosis with a lower chance of recurrence after surgery. We also discovered a new cluster in a subsequent analysis of colorectal cancer [2]. Further detailed analysis revealed the population characteristics of cancer cells and their relationship with the microenvironment, such as immunity, vary greatly among clusters. Cancer cells are abnormal versions of our own cells which can be recognized as non-self, and therefore be eliminated by the immune system. However, selective pressures can drive cancer cells to change and evade elimination. The individuality of the cancer cells and the microenvironment, as well as the treatment implemented, will affect the future development of the tumor. For most cancers, the higher the immune activity, the better the prognosis. In surprising contrast, for cancers in immune-privileged sites, such as uveal melanoma and low-grade gliomas, we found that high immune activity is associated with worse overall survival (Figure 1-2), and we elucidated the mechanism [3]. We aim to clarify the mechanisms that cause these differences through the analysis of omics and pathological images, and to create a model for predicting the effects of treatment using mathematical simulations, with the aim of providing precision medicine that allows treatment to be optimized for each patient.
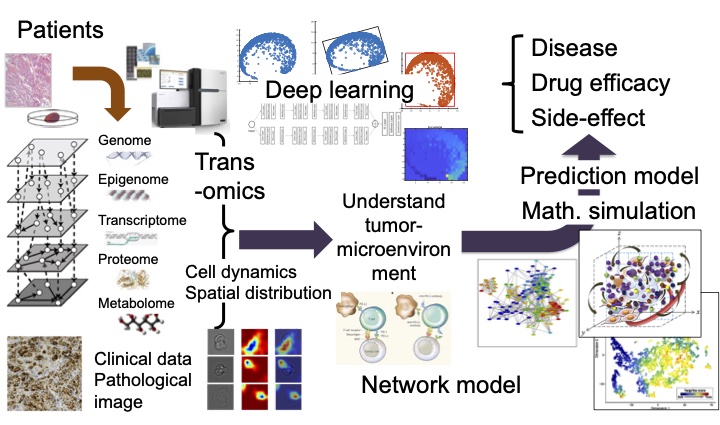
Figure 1-1. Unravel and predict diseases with patients’ omics, clinical, and pathological image data.
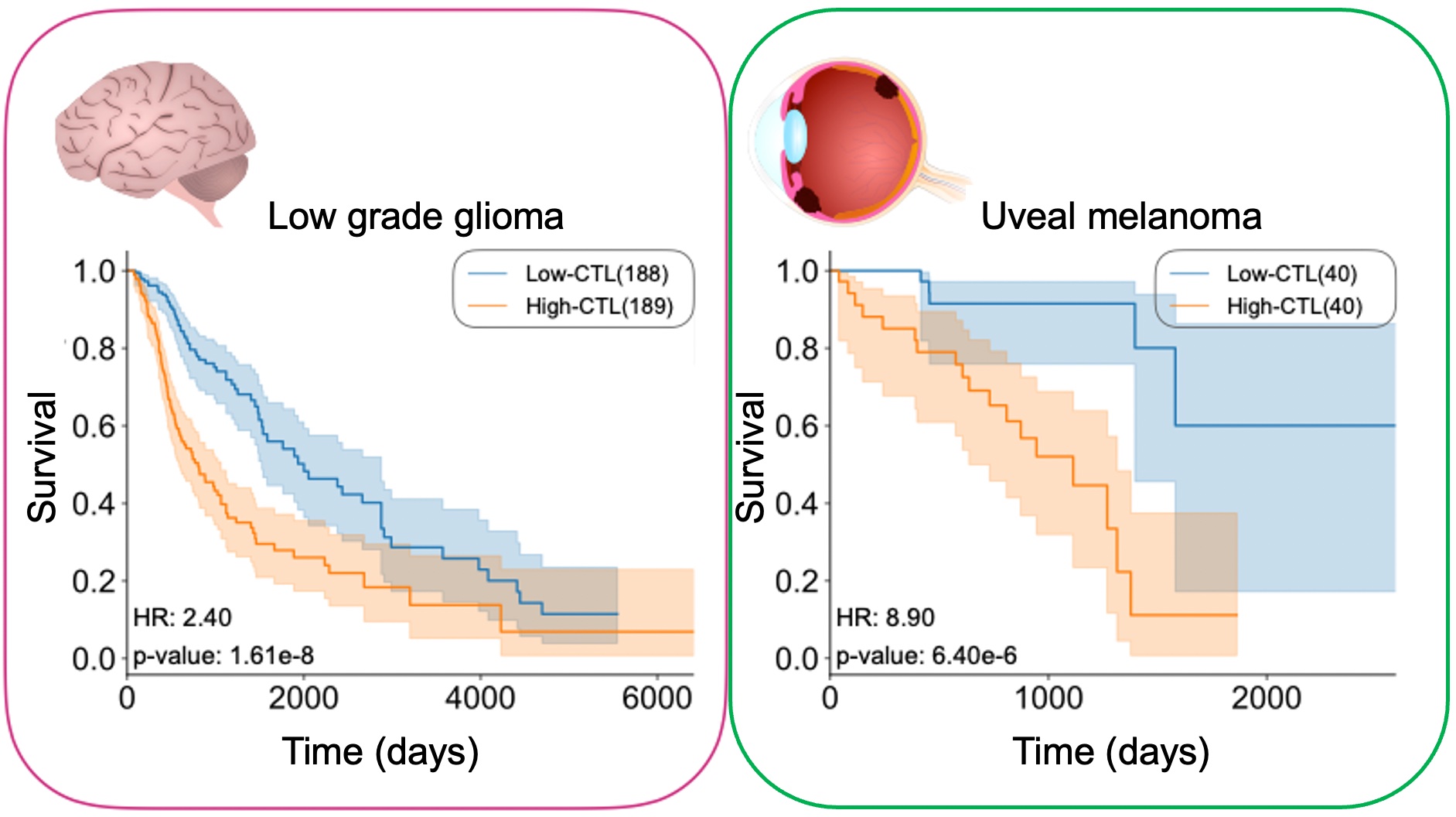
Figure 1-2. Cancer types in which higher the immune activity corresponds to worse overall survival.
Methodology for original analysis with deep learning
We research the ability of convolutional neural networks to process not only images, but also non-image data, particularly omics data. We developed a unique technique “DeepInsight” for transforming omics data to look like an image and applying convolutional neural networks to it, so that we can distinguish cancer types from omic data (Figure 2-1) [4]. We also developed the technique “DeepFeature” which analyzes the inner workings of neural network to identify how it discriminates between cancers (Figure 2-2) [5]. Through these techniques, we discovered a new signaling system that indicates the individuality of cancer types. In other words, we were able to show that deep learning can be used to make new scientific discoveries.
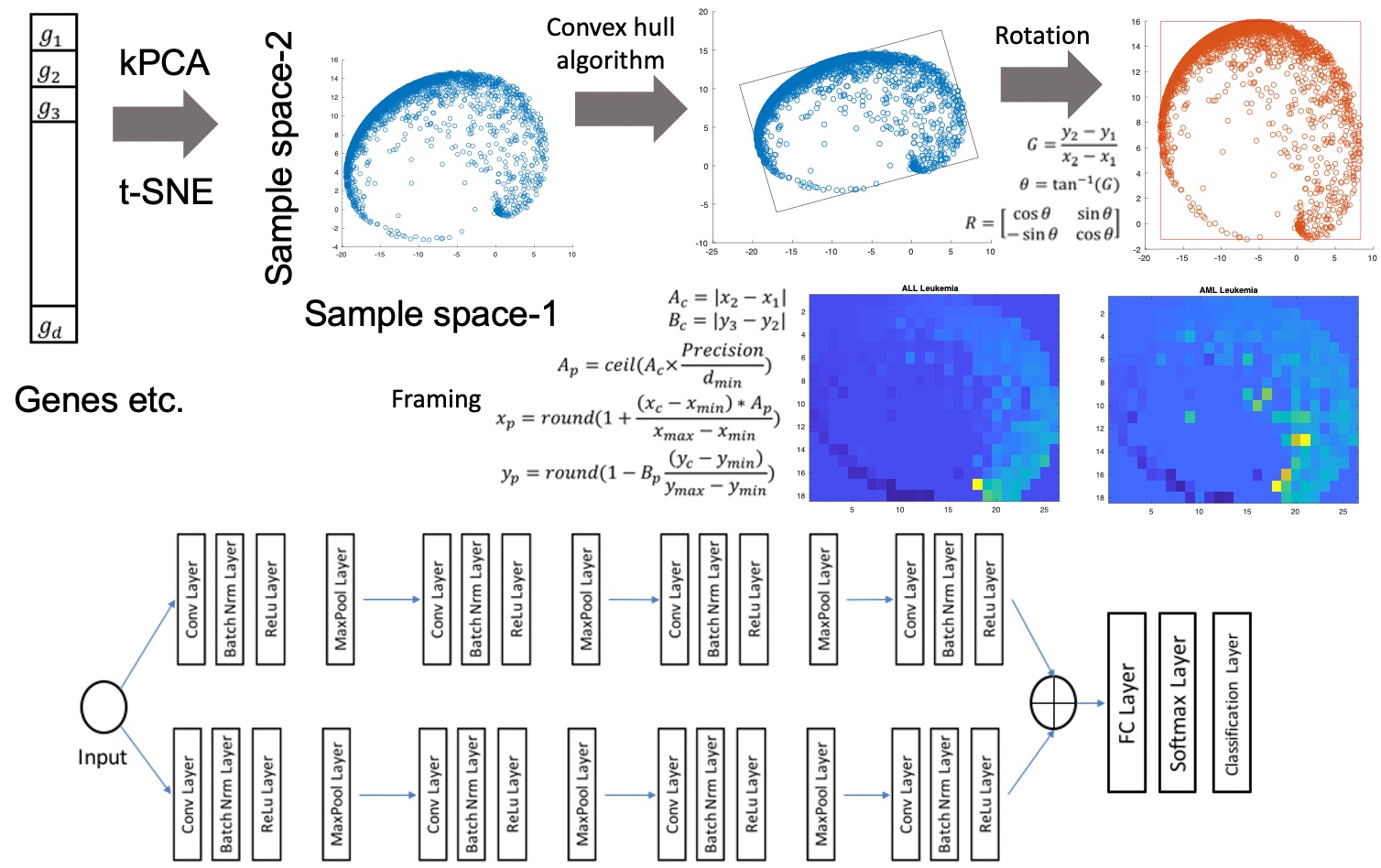
Figure 2-1. Our new technique that converts omics into images and inputs them into deep learning [4].

Figure 2-2. Extracting features important for differentiating cancer types [5].
Prediction of anticancer drug response by multi-omics and deep learning
We further advanced our DeepInsight method and proposed DeepInsight-3D, a new method using deep learning to predict patient-specific responses to anticancer drugs from multi-omics data (Figure 3-1) [6]. Along with significantly better prediction performance than conventional methods, we discovered gene patterns (Figure 3-2) and new pathways that differentiate responses to anticancer drugs.
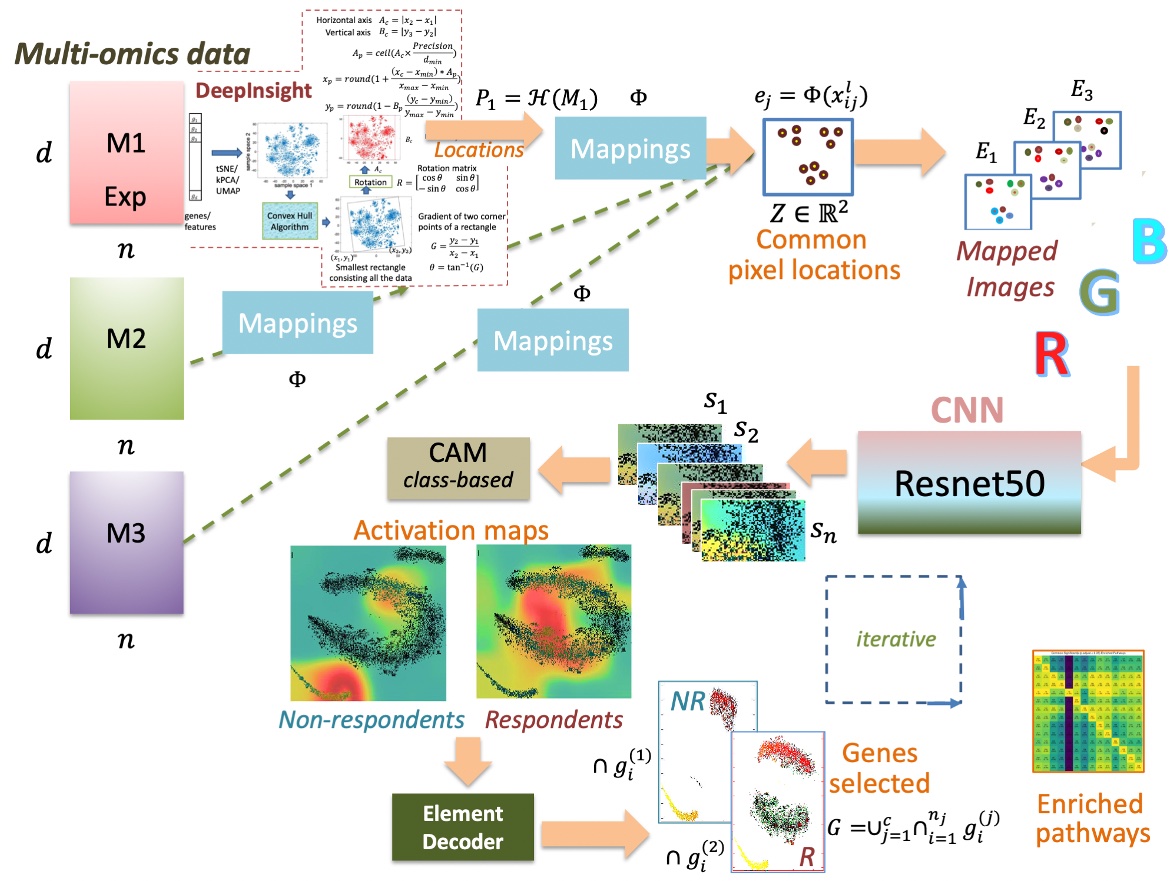
Figure 3-1. Prediction of anticancer drug response by multi-omics and deep learning [6].
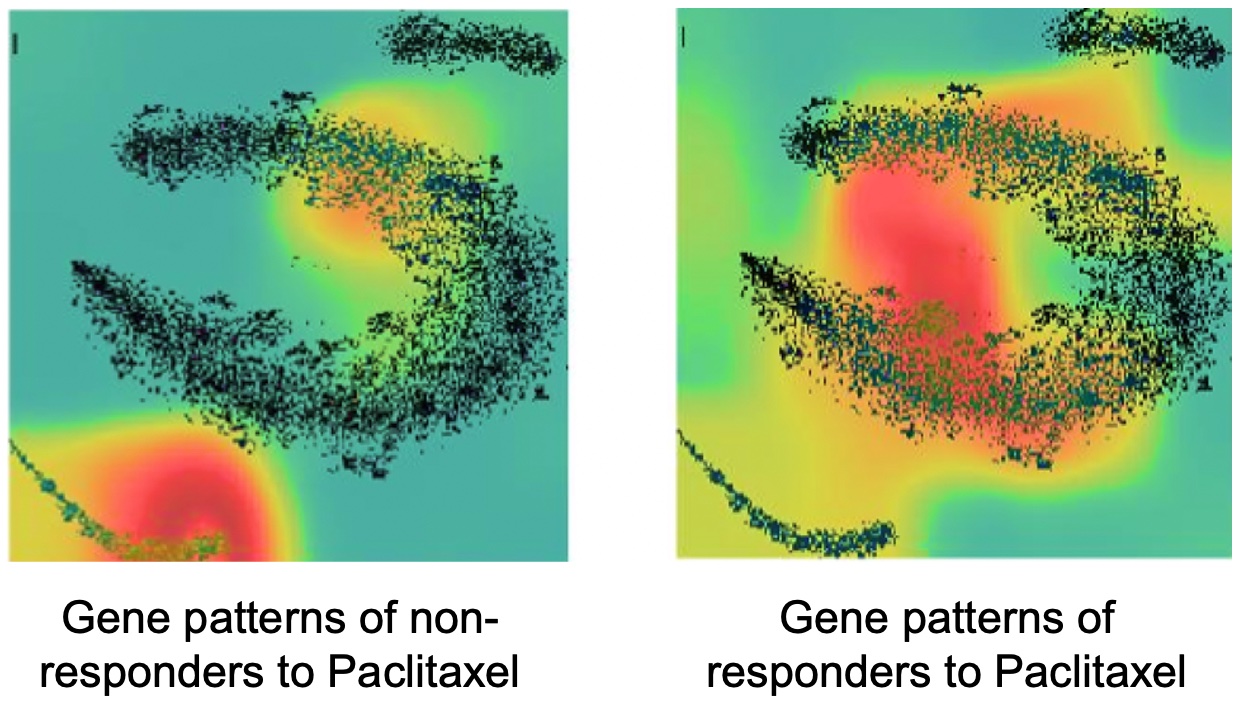
Figure 3-2. Differential gene patterns involved in non-response (left) and response (right) to the anticancer drug paclitaxel.
Deep learning to identify cell types for single-cell RNA-seq data
Based on our DeepInsight methodology, we proposed scDeepInsight (Figure 4-1), a new method using deep learning to identify the original cell type from single-cell RNA-seq (scRNA-seq) data [7]. Cell type identification is key to studying cell population heterogeneity through the analysis of scRNA-seq data. This method utilizes convolutional neural networks (CNNs), which have high image classification and feature extraction capabilities, by transforming scRNA-seq non-image data into images using our originally proposed DeepInsight method. As a result, scDeepInsight was able to identify cell types with much higher accuracy than other state-of-the-art methods (Figure 4-2). The proposed method is expected to make a broad contribution to future research related to the elucidation of the mechanisms of cell regulation in vivo and in disease using scRNA-seq data.
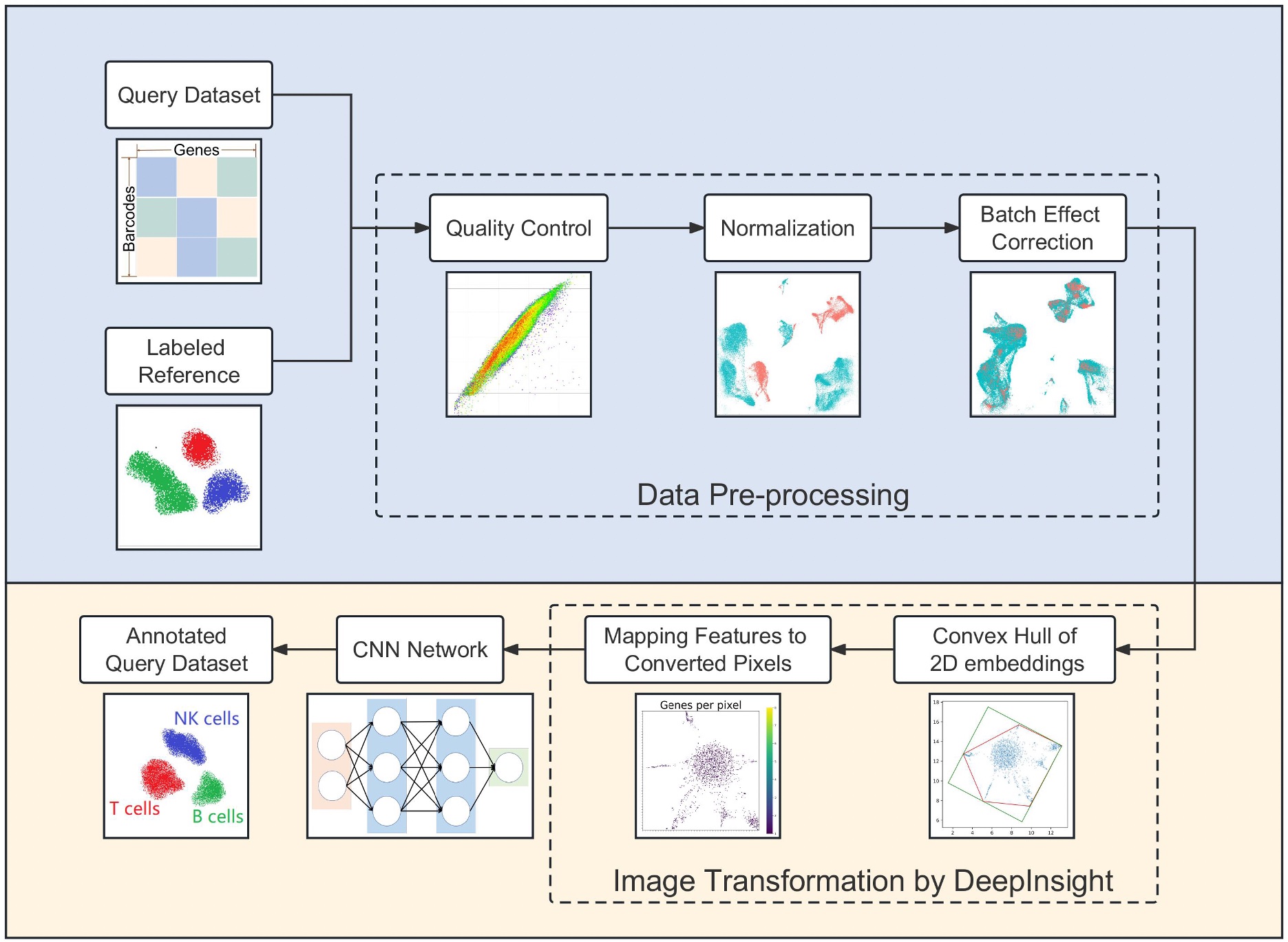
Figure 4-1. scDeepInsight Pipeline.
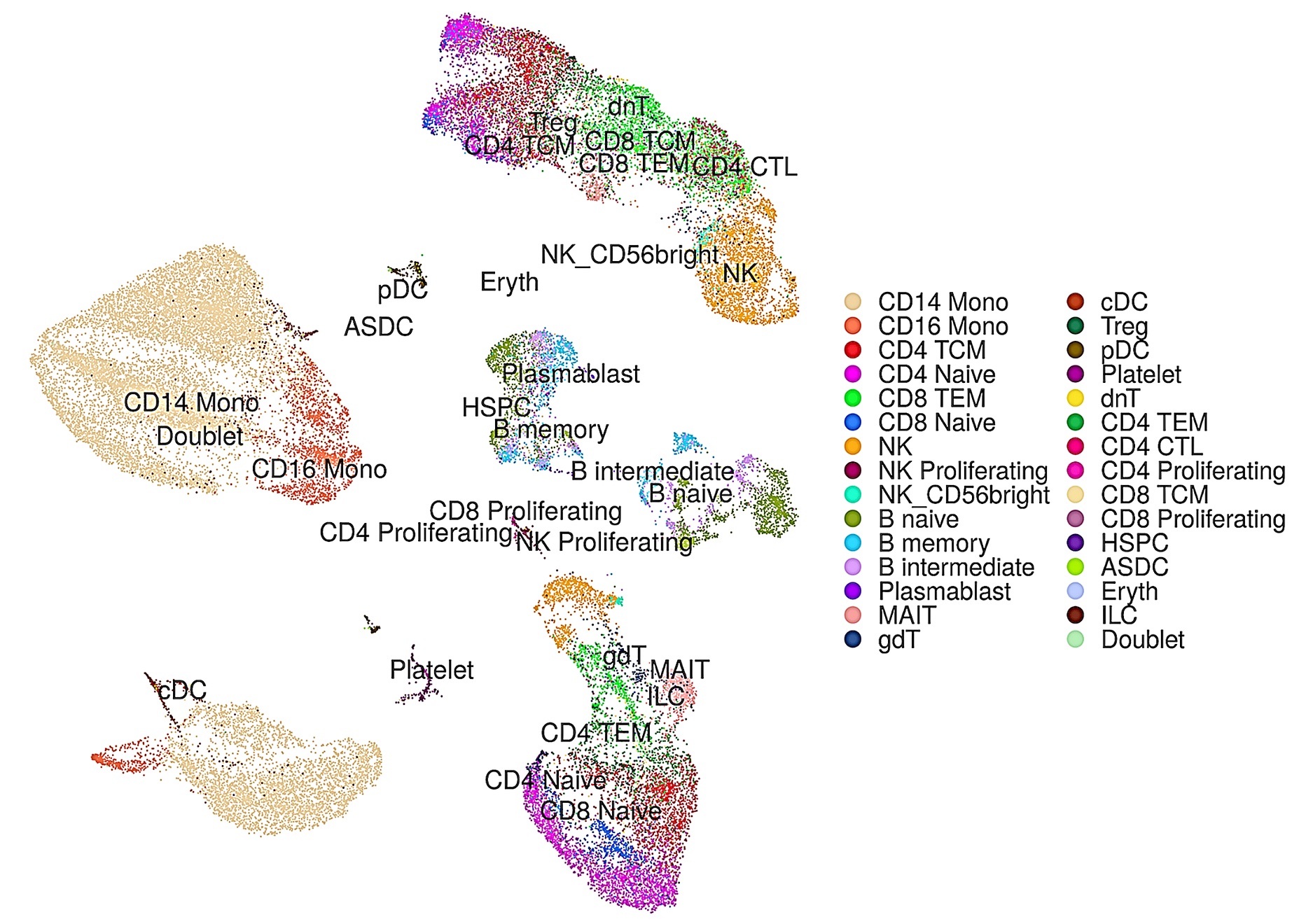
Figure 4-2. Color-coded results for each cell type predicted by scDeepInsight.
Various people gather and research
Our laboratory is located at Hongo campus. In addition to our research projects mentioned above and of quantum computing, we are developing methodologies to identify and sequence molecules by analyzing signals from our originally fabricated nanopores in collaboration with the Uemura laboratory of the department of biological sciences, school of science, the University of Tokyo, as well as many collaborative research projects with medical institutions. Our lab includes bioinformatics researchers, clinical doctors, genetics researchers, sequence analysis staff, network analysis researchers, and mathematics researchers from Japan and abroad, sharing their expertise and knowledge to further our research every day. Our laboratory promotes medical science with an emphasis more on analytical methodologies than experimentation.
References:
[1] Fujimoto A, Tsunoda T, et al. Whole genome mutational landscape and characterization of non-coding and structural mutations in liver cancer. Nature Genetics, 48, 500-509 (2016). [2] Sugawara T, Miya F, Ishikawa T, Lysenko A, Nishino J, Kamatani T, Takemoto A, Boroevich KA, Kakimi K, Kinugasa Y, Tanabe M, Tsunoda T. Immune subtypes and neoantigen-related immune evasion in advanced colorectal cancer. iScience, 25, 1003740 (2022). [3] Matsuo H, Kamatani T, Hamba Y, Boroevich KA, Tsunoda T. Association between high immune activity and worse prognosis in uveal melanoma and low-grade glioma in TCGA transcriptomic data. BMC Genomics, 23, 351 (2022). [4] Sharma A, Vans E, Shigemizu D, Boroevich KA, Tsunoda T. DeepInsight: A methodology to transform a non-image data to an image for convolution neural network architecture. Scientific Reports, 9, 11399 (2019). [5] Sharma A, Lysenko A, Boroevich KA, Vans E, Tsunoda T. DeepFeature: feature selection in nonimage data using convolutional neural network. Briefings in Bioinformatics, 22, bbab297 (2021). [6] Sharma A, Lysenko A, Boroevich KA, Tsunoda T. DeepInsight-3D architecture for anti-cancer drug response prediction with deep-learning on multi-omics. Scientific Reports, 13, 2483 (2023). [7] Jia S, Lysenko A, Boroevich KA, Sharma A, Tsunoda T. scDeepInsight: a supervised cell-type identification method for scRNA-seq data with deep learning. Briefings in Bioinformatics, 24, bbad266 (2023).